Sensor data in Industry 4.0: a change of reference in the optimization and maintenance of machines and systems, and a source of recurring income with the creation of new customer services.
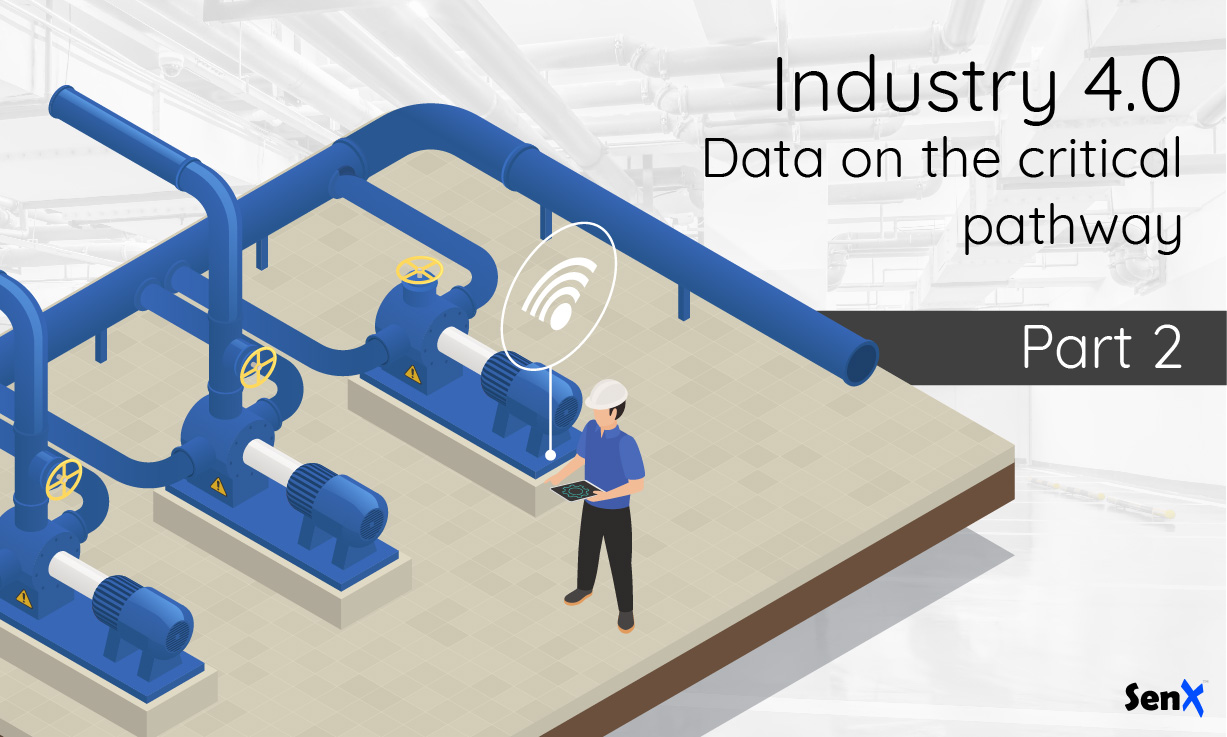
This is the second part of a three-part series on the industry of the future and how data will play a major role in the transformation of companies in this sector.
Didn't read the first part? It's over here.
Vous préférez lire cet article en français ? C'est par ici.
Sensor data to optimise system operation
The operational requirement implies that systems must work towards zero defects and total availability.
The interdependencies and interlocking of systems are such that the failure of one of them can lead to a chain of effects, which can quickly have serious financial consequences. This state of affairs makes maintenance critical, with the cost remaining a constant concern for companies. The ratio between the total costs of maintenance and repair and the amount of the corresponding investments (a ratio often used), is on average 7% of the initial amount of the investments, although it can vary greatly from one industry to another.
There are three models of maintenance that are traditionally used by companies:
- Reactive maintenance. It limits recurrent costs and can be adapted to equipment whose probability of failure is considered very low. The counterpart of limiting recurrent costs is taking a risk, the consequences of which can be serious, if necessary.
- Conditional maintenance. This is based on continuous monitoring of a system's behaviour with a view to triggering maintenance actions when it seems most appropriate. The difficulty then lies in correctly managing the criteria for deciding when to stop a piece of equipment. All too often, operational pressure is such that, in practice, condition-based maintenance slips into reactive maintenance.
- And preventive maintenance. It involves both immobilising equipment and having a stock of spare components and dedicated teams. The latter is practised in particular in highly regulated sectors, such as aeronautics or nuclear power, for example.
The first two forms of maintenance are based on measurements from sensors. They are used in real-time through thresholds such as: normal value, value to be monitored, abnormal value (alarm), dangerous value (critical alarm). As for preventive maintenance, often perceived as costly, it is based on asset management. It is usually supplemented by conditional maintenance.
Analytical Maintenance as an intermediate way to Industry 4.0
The analytical exploitation of measurement history opens up an intermediate path, with the increasing number of sensors providing a potentially exhaustive understanding of the functioning of a piece of equipment down to the smallest detail. It is necessary to have relevant and efficient sensors that transmit a sufficient number of measurements. We then need high-performance technical tools that are capable of storing sufficiently deep historical data and analyzing it, if necessary in large quantities, during production.
This intermediate path is that of Analytical Maintenance, based on the analysis of time series of data, with a preference in passing for a broader terminology than that of predictive maintenance. Maintenance Analytics covers four types of analysis: descriptive analysis, diagnosis, predictive analysis, and prescriptive analysis.
Its advantages
In terms of direct costs, it is between conditional maintenance and preventive maintenance.
Diagram showing the impact of direct costs (repair costs and recurrent Capex + Opex) according to the types of maintenance
But direct costs are not the only ones to be taken into account, as illustrated by the following two charts:
The first of these charts shows the financial impact of equipment downtime due to planned preventive maintenance or due to a breakdown.
In both cases, analytical maintenance can reduce the financial impact. Deloitte estimates that Predictive Maintenance alone can reduce the time spent on maintenance by 20 to 50% while increasing equipment uptime by 10 to 20%. McKinsey offers similar figures: a 20-30% reduction in maintenance costs and a 20-50% reduction in downtime. It also emphasizes organizational resistance.
Analytical maintenance also reduces the indirect negative effects of equipment downtime in terms of potential disruption of supply and sales. A machine or system is part of a chain that, due to the failure of a single link, can come to a complete standstill.
The repercussions can then have serious consequences upstream when supplies have to be suspended. But also downstream, with the suspension of customer deliveries and their possible commercial consequences (penalties, loss of customer confidence, loss of new contracts). The resulting indirect costs are probably the most important and are also the most difficult to estimate.
In fact, there is little doubt about the benefit of analytical maintenance in terms of maintenance costs to the company.
However, it is still necessary to have powerful and industrializable tools that are able to process the data while interacting with the company's information system and therefore with the various ERP modules as we saw in the first article. This is Warp 10's ambition in the most varied environments with potentially numerous and varied sensor data, each with different behaviors.
Sensor data to transform the customer relationship
The most important transformation is complementary to the one affecting maintenance. It is linked to the new uses that are gradually being made of technical data. Whereas technical data used to have only an operational value linked to the functioning of equipment, machines, and other systems, it is gradually becoming the stake in the transformation of the relationship between a supplier and its customer.
This orientation is not new in areas such as energy or mobility. Data was originally used to ensure and optimize the technical functioning of networks, buses, and cars. It is now of interest to the user as well as to the many service providers who can offer him new services. As a result, the segmentation of the market has become more complex, with the need for each player – whether it wants to maintain its leadership or wishes to position itself as a new entrant in the value chain – to control the data. And above all, the data it generates through its own systems and equipment.
The emergence of new services
Even if the framework and the modalities differ, the industry is following the same path, in different forms depending on the sector. The focus of this evolution is a profound transformation of the profession that every industrialist could be confronted with if it is not already the case: the transformation of an industrial product into a service.
We illustrate this with an example below.
Someflu is a manufacturer of industrial pumps, in particular anti-corrosive centrifugal pumps, designed to convey various corrosive or abrasive fluids.
The maintenance of these pumps is very important because of the products involved and the operational or safety constraints on the industrial users. At the same time, the manufacturer operates in an internationally competitive environment where the selling price plays a key role.
Alexandre Lacour, Someflu's director, realized that processing sensor data offered him the opportunity to change his model. By choosing to systematize their use, he has given himself, among other examples, the possibility of eventually :
- to carry out exhaustive tests at the end of production in a systematic manner ;
- to have a real-time view of all the equipment by customer;
- compare systems and detect discordant operation of equipment;
- analyse the ageing of each piece of equipment or family of equipment;
- predict potential failures;
- schedule maintenance operations for the actual condition of equipment parts.
It can also, and above all, allow its customers to benefit from this exhaustive vision of their equipment. New services made available via the Internet or directly on mobile phones can be developed for this purpose. All this with links to its own information system. These services, which can be invoiced, constitute a new source of revenue for the manufacturer; they are also recurrent, which is likely to profoundly modify the nature of the relationship with its customer.
Thus, Someflu acquires the ability to change the pricing of its equipment by adjusting the balance between the selling price and the service price. In the end, the selling price can be, if necessary, zero since it is integrated into the overall service cost.
SenX is supporting Someflu in this transformation with a recovery of sensor data and, thanks to Warp 10, an advanced analysis that allows it to aim for a change in its business model. Its director, Alexandre Lacour, is now involved in the French Industry of the Future inititative with a vision that is precisely the one highlighted throughout this article. This vision is described very well in an online video that is particularly enlightening.
SCADAs and digital twins
The last article in this series will deal with the SCADAs and one of the hottest topics in Industry 4.0, namely the digital twin.
Follow SenX and Warp 10 on Twitter for future blog posts.
The end of this article in part 3.
Discover the different stages of maturity of Industry 4.0 in the video below. (In French, English subtitles available)
Read more
Amzair: A 10-year warranty, thanks to IoT
Introducing FLoWS, a functional language for Time Series Analytics
Les données d’énergie à l’aune du déconfinement
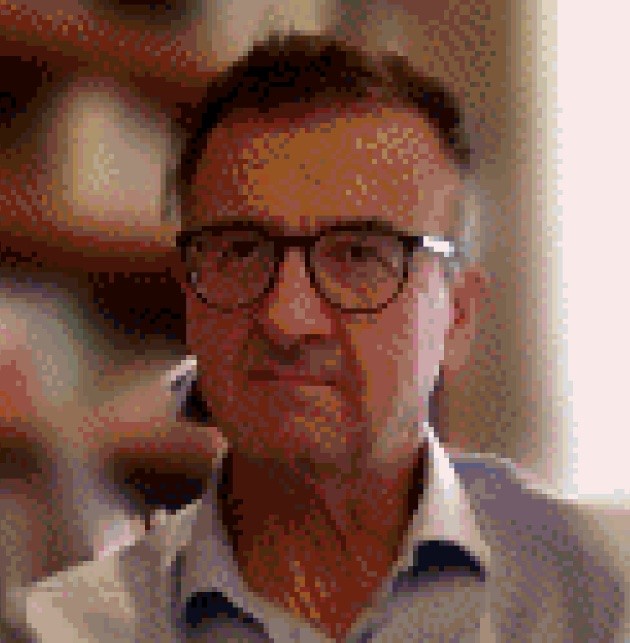
Co-Founder & former CEO of SenX