We discuss about how information systems are historically handled, and why time series databases will play a critical role for data in the near future.
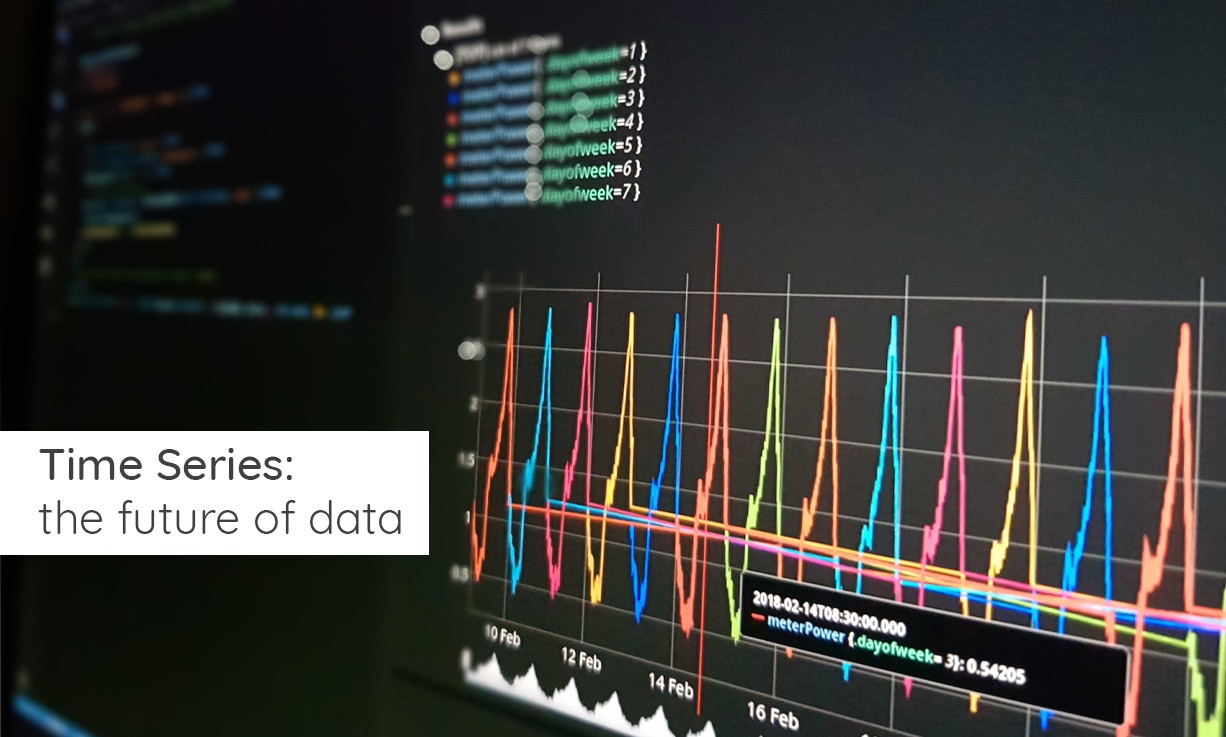
To address the data processing needs of the world of sensors and the Internet of things, SenX has developed Warp 10 and WarpScript, a suite of software based on data models known as Time Series and Geo Time Series. In this article, we dive into the historical reasons why the industry is shifting toward time series models.
In order to cope with these demanding needs, organizations must transform the way they think about their information systems. Only by doing so will they be able to compete with other major digital players in the world. It is the real challenge that is underlying. That is why the "all-connected" world where we live is witnessing the advent of time series databases.
Learn more about What is a Time Series Database. |
Diversity of data types and their processing
Within companies, data is generally managed in large "relational" databases, also known as structured or SQL databases. Derived from business applications, they are structured with strong functional dominance. Formats and semantics within these databases are specific to each sector according to its needs. Mostly, every company uses relational databases, which are managed by their IT department (or an external company).
Until the mid-2000s, the question of changing the data model did not arise. Any new project would naturally fit in an existing mold.
Data explosion
The growth of messaging and social media has changed the situation. Soon, the volumes of data (texts, sounds, images, videos) became considerable while avoiding the constraints of business applications. With this in mind, the major players on the Internet (mainly Google and Facebook) had to imagine other database structures: NoSQL databases.
The rise of sensors, and more generally of the Internet of Things, has created other constraints. They generate volumes of data with a very variable frequency in each case. We are able to process data that can be transmitted thousands of times per second.
But we also need to cope with numerous sensors which emit flows in parallel with different characteristics. In addition to a massive data processing capacity, it is necessary to analyze the considerable flows of raw information to be able to derive value.

This set of constraints has led to the emergence of a particular model of data: time series
They are already known, in a special format, to economic statisticians. It is no longer a question of organizing data according to functional criteria linked to business types, but to focus on their temporal occurrence. The main input key of the datum is its timestamp, with accuracy and synchronization which should be as close to perfect as possible.
To these different types of data (structured, unstructured, temporal) we must add a fourth one. This one covers graphical representation in two or three dimensions. For example, geographic information systems, 3D modeling, video games, virtual worlds, augmented reality… Ultimately, four families of data are translated into different technologies and four visions that have little in common.
Faced with strong growth in data volumes, all players claim to be doing "Big Data". However, we use this terminology, now popularized by the media, to refer to very different realities.
Moving away from IT silos
In typical information systems, applications and professional services produce structured and formatted data according to business specifics. Each sector of activity has its modes of representation and semantics.
Relational databases have adapted to data manipulation requirements. Their structure makes it possible to confront two essential constraints:
How information systems are historically handled, and why time series databases will play a critical role for data in the near future. Share on X- Manage the links between different types of data. It facilitates the development of business applications (purchases, sales, financial movements, operations management, etc.)
- Guarantee the integrity of the data. Mechanisms guarantee the return to the initial state in the event of a problem in the middle of an operation.
Data silos architecture
This organization of business lines has led to data silos architecture. It has dependency links between business needs, applications that respond to them, and data that stem from them. The German SAP group markets the world’s most widely used software suite. They have many modules structured within an ERP.
Then, Business Intelligence (BI) has considered suspending silo-based information systems. They begin to extract value from data coming from one or more business applications in a transversal manner, at great expense.
For example, costly (and sometimes sophisticated) mechanisms (ETL) for extracting data into data warehouses have been implemented to retrieve data. Those data can be cross-referenced (e.g. online consultations by customers with their purchases, after-sales interventions …). These tools have made the success and fortune of companies like Business Objects, SAP, or SAS.
This two-tiered computing with a main (business) function and a secondary(BI) function is bound to disappear. The idea is that the data will have multiple lives and multiple uses, and that information systems must be organized accordingly.
The organization of data in silos that is still predominant today is not suited to this evolution.
Issues associated with relational architectures
The use of data for multiple purposes is subject to several barriers in classical architectures:
This is the reality of traditional information systems. They are based on relational databases suited to so-called transactional applications (e.g. updating a customer account, a stock, etc.). However, these transactional data will soon represent less than 5% of the total volume of data that companies process.
Time series, a disruptive model for the organization of data
Initially intended for sensor data, time series databases are a subset of non-relational databases. They are "column-oriented" whereas relational databases are "line-oriented". Without going into technical details, we will remember that column-oriented databases offer advantages.
For example, it allows a better adaptation to data compression and the easy addition of new data sources. It also optimizes the use of storage disk access by decreasing the need for memory which cost can become prohibitive, parallelization of computing jobs on several servers…
The most used column-oriented technology to date is HBase, a subproject of a group popularized under the name of Hadoop. This set constitutes an ecosystem that turns information systems into a new world made up of new approaches and tools.
Data modelled with series
With time (or geo time) series, the idea is that many data, beyond sensor data, could be modeled with series. Indeed, we can approach any business activity as a succession of unitary events in time and space.
Therefore, rather than focusing on the business sense of the data (with its object, its format, its semantics …) resulting from an activity, we should consider that the first structure of the data lies in its precise date of emission. And optionally, its location.
In doing so, any process becomes a succession of events or micro-events that generate data which are as many time series or Geo Time Series.
By relating all data back to time (and geolocation when available), it becomes possible to cross many and heterogeneous data streams in a much easier way than in conventional information systems.
More generally, the five barriers mentioned above can be overcome much more easily:
This evolution is the one that represents the migration of a world of transactions towards a world of flows.
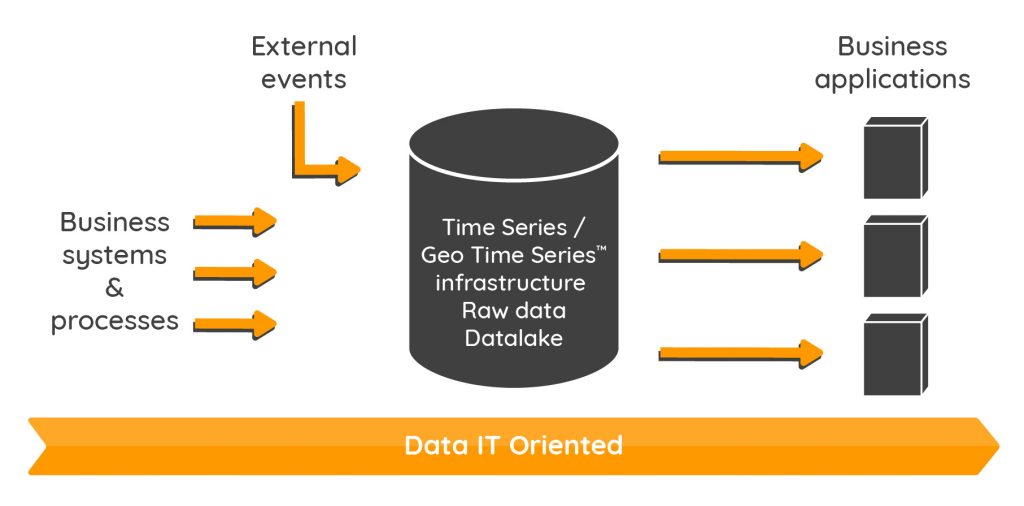
The architecture of two-tiered information systems is being erased in favor of a data hub (the datalake). It will aggregate all event measurements and will feed applications such as business applications, financial applications, marketing … or others.
Application use cases
Among the most advanced business sectors that are familiar with Time Series, we can point out the following ones:
- Industry. Industry 4.0 becomes a reality and any systems and components have to be monitored. Sensors are spreading in all levels of manufacturing and also in logistic and in products themselves,
- Aeronautics. New aircraft are equipped with several hundred thousand sensors, some of which produce hundreds of measurements per second,
- Energy. The sector faces the growth of renewable energies and consumption challenges. All the chain requires real-time balancing between a large range of systems and components,
- Mobility. From traffic management to connected cars and Mobility as a Service, real-time data become the key asset to develop new services for professionals and for final users,
- Telecommunications. Mobiles produce a huge amount of data, and they host applications that generate their own data. The smartphone is a kind of ubiquitous sensor, including the benefit of new services for users and the risks related to the use of personal data,
- IT Infrastructures. A typical use case for Time Series that is used for IT Monitoring, including predictive maintenance,
- Smart Cities. All infrastructures take profit of sensors: mobility, car parks, energy, waste, security, building, mobile applications… and data has to be cross-referenced progressively (energy/building, energy/mobility, mobility/security …),
- Health. Beyond legacy IT management, data include health data from patients and from health monitoring systems. All data are very sensible and many of them are sequenced data.
- Defense. Real-time data has always been of particular importance in defense systems, information and command systems, security, and cybersecurity. The new generations of sensors give a new dimension to the data issue.
We would have to add other sectors like logistics, insurance, banking, agriculture, sport …
Data engineering and Time Series
Many companies and public organizations are still carrying out an exploratory phase regarding the potential of their own data. That is particularly the case for sensors / IoT data. In this phase, the Data Scientist is the key people that can address business use case needs by implementing data modeling, data analytics, data visualization… The Data Scientist seems to be the providential prophet that promises a profitable future and that everybody's tearing out.
However, there is a major gap between a use case proof of concept and operational business processes. The constraints related to the real world mean an increasing range of sources of data including their own format, rate, variations… and security issues. Datalakes cannot be defined use case by use cases. They require a strong data engineering approach and expertise.
Data Science and Data Engineering are the two legs of any genuine data strategy. They have to combine, to interact, to challenge in order to address business needs. A data scientist is not an architect, and a data engineer is not the one who can properly translate a business need into software code. An O'Reilly's paper highlighting the differences between the two approaches and profiles will be read with interest.
The lack of data engineering expertise in organizations is probably one of the key reasons that explain the tricky challenge to move from POCs to the industrialization of data analytics services.
SenX's vision
SenX proposes an original vision on the future of data by considering that most of the operational data refer to sequenced data. That means that most of the operational data are Time Series (or Geo Time Series). It is a perspective based on the assessment that the volume of sequenced data including data from IoT, sensors, systems, processes, human actions, environment events… is going to become prominent within organizations. Except for descriptive static data, what else than the timestamp as a kind of universal index?
Warp 10 is precisely a solution that addresses this vision from an industrial perspective including performance, scalability, interoperability, and security.
To conclude
Starting from this paper, we are going to explore strategic approaches in different vertical businesses: Smart Cities, Mobility, Energy, Industry, Health… Each of the sectors will give rise to a dedicated article.
In the case you are looking for any information for a specific sector, please contact us at contact@senx.io
Read more
Our vision of Industry 4.0 and the 4 stages of maturity
Industry 4.0: Data on the critical pathway (1/3)
Les données dynamiques au cœur des Smart cities
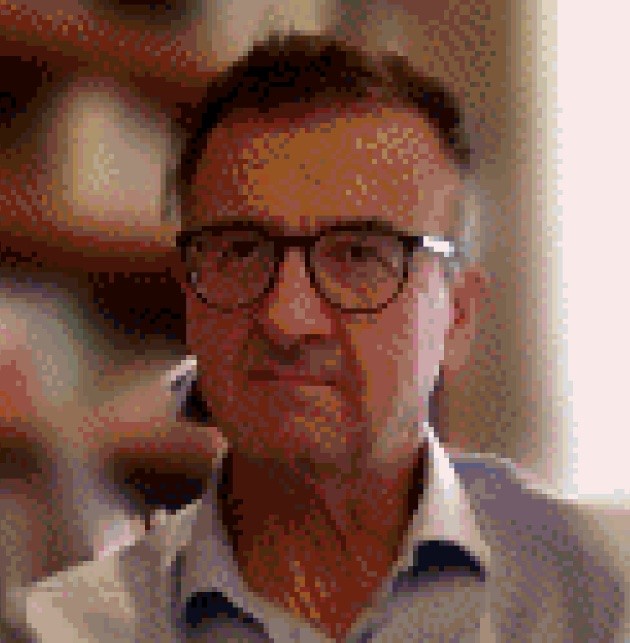
Co-Founder & former CEO of SenX