Using AI to make industrial assets more efficient and reduce their downtime is on many agendas. Learn how digital twins and time series data play a major role in this plan.
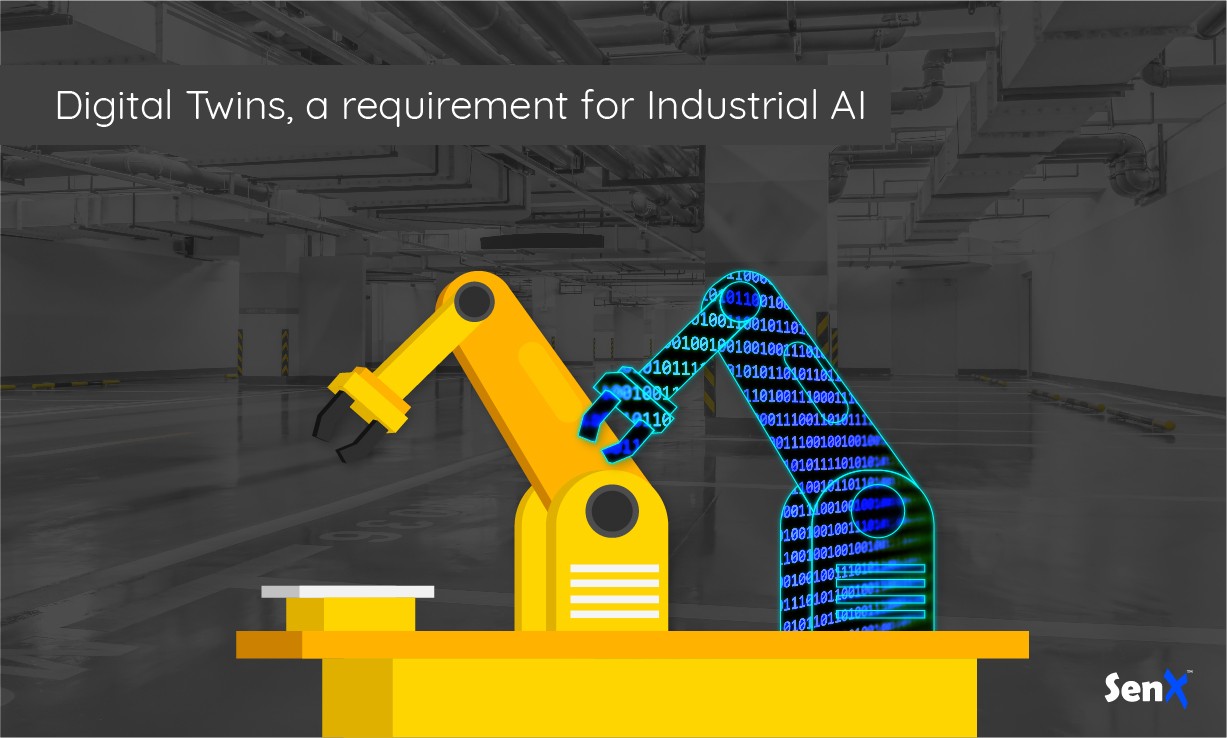
When interviewed, CEOs across industries all state that AI is part of their top priorities. But when it comes to actual implementation AI projects are not very glamorous. Past simple proofs of concept and the hiring of a team of data scientists, there is usually no sign of the highly anticipated digital transformation wished by the CEOs.
There are multiple reasons for this disenchantment, far too many to list here. But among those, some are directly related to what we focus on at SenX, data, and the way industries introduce them in their environment.
No digital transformation without data
The willingness to transform is genuine in many organizations, driven by ambitious visions or just the consciousness that the competitive landscape is evolving.
The next step is usually for those businesses to pick some quick wins to prove that the transformation can be initiated and comfort everyone that it does not mean changing teams or radically modifying their way of working.
Those short projects aim at demonstrating the methodology for transforming limited operational perimeters. They often involve solving a problem with approaches to leveraging new technologies. Those technologies, 100% digital, need fuel to work, and that fuel is data. So the first step is to ensure data are available.
The firms hired helping in building those quick wins will then wander among departments. They will harvest datasets here and there until they have sufficient matter for implementing their solutions.
This step can sometimes take time if the data is not well identified and distributed across the organization. But it is a mandatory path to follow as without data no digital transformation will happen.
No AI without big data
Past the simple quick wins done to bootstrap the transformation comes a time when more ambitious projects are brought to the table, and that is when AI (Artificial Intelligence) comes into the conversation. The hype around AI is so strong that projects around AI and ML (Machine Learning) cannot be neglected.
The problem with the current hype is that very few people really understand what AI actually implies. For many, you buy an AI like you buy a Microsoft Office 365 subscription, this is just not true. The promise of AI is to bring new, automatic, ways to use data to help in or even completely assume the decision process. This promise can only be fulfilled if the actual AI put to work, otherwise called the model, is actually trained on the data in your very own organization, and this requires once again the same digital transformation fuel, data. The difference is that this time you need more of it. You are no longer trying to light a fondue burner but a rocket engine!
Training a model does indeed require a lot of data covering the various aspects of your business operations you want the model to focus on, also covering a long period of time so trend and seasonality can be modeled.
This has several impacts
- The first is that you cannot expect to train a model and efficiently introduce AI in your operations until you actually have collected enough meaningful data. And if your organization has not done so far you need to start as soon as possible.
- The second impact is that this data collection process is not a one time job. It does not stop once you have enough data for training a model. It needs to go on and on, so you keep on accumulating signals on how your business operates to retrain your models in the future if their performance starts to degrade. This means that prior to your journey into the core of AI you need to plan for big data to be collected, stored and made available to teams across your organization, so they can start looking at the data and imagine possible uses and models.
No industrial AI without Digital Twins
Among verticals, industrial organizations face the hardest problems of data collection. Industries whose data mainly relates to users using their services are lucky. In the end, their data are not that massive. Sure we have all heard stories of banks or retailers hoarding piles of data. But we are talking about a few thousand interactions per year per user. So even with a billion users, which not that many banks or retailers have, we are talking a few trillion events per year.
In the industrial world, things are different, the assets producing data do not eat or sleep. They work day and night and sometimes produce thousands of measurements per second.
For example…
Take for example the CERN experiments at the LHC. They produced 600 million events per second during the campaigns for the quest of the Higgs boson. That is 51 trillion events per day. Luckily for the CERN, not all events needed to be retained. With highly efficient AI-based detectors, which needed to be trained with massive data themselves, they were able to limit the production to 100 000 events per second sent for digital reconstruction and ultimately 200 events persisted per second.
But other sectors need to retain more data. Synchro phasors (or PMUs, phase monitoring units) monitoring electrical grids, for example. They each produce several 1000s measures per second, and there are thousands of those at the scale of a country like France. This means millions of C37.118.2 messages sent every second, not to mention the IEC61850 messages sent to supervise the substations.
Learn how time series data allow creating digital twins of assets and play a major role in enabling the use of AI in industry. Share on XSame thing in aeronautics where aircraft typically produce 5 000 to 15 000 data points per second they are operating, or industrial assets whose PLC (Programmable Logic Controllers) track the state of many sensors and actuators.
The use of AI in those verticals requires that those truly massive data be collected and organized. Since they are data related to physical assets, it is wise to use an approach which mimics these assets in a digital form, this approach is called Digital Twins.
What are Digital Twins?
The Digital Twin of an asset is the set of measures coming from its sensors and actuators. Those measures need to be tracked in time to catch the dynamics of the assets' operations. And the technology of choice to do so is a Time Series Database. Indeed, Digital Twins are nothing else than time series, some for the sensors, some for the actuators with their states. And if you want more advanced digital twins, some with the control commands sent to the assets to modify how it behaves.
Once you start collecting the data from your assets in a Time Series Database, you can easily access the state of those assets at any point in time. More importantly, you can start extracting features to train models to detect anomalies and perform predictive maintenance.

Takeaways
AI is on every business' agenda, but the importance of data is too often overlooked. When it comes to industrial AI, the first step towards a successful implementation is the collection of all sensor data to build Digital Twins of the physical assets involved. This approach needs to leverage a Time Series Database, the kind of database SenX offers with the Warp 10 Time Series Platform.
Contact us to learn how SenX and its technologies can help you master your industrial AI adventure.
Read more
Our vision of Industry 4.0 and the 4 stages of maturity
Building a Data Historian based on OPC UA, Warp 10, and Telegraf
Demystifying the use of the Parquet file format for time series
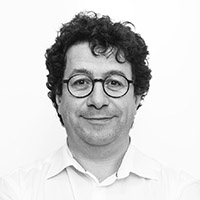
Co-Founder & Chief Technology Officer